Precision Livestock Farming: New information to help decision-making? (Full text available in English)
Header
New techniques known collectively as “precision livestock farming” are flourishing. They provide farmers with a profusion of data and information, some of it new. However, it is not clear whether the information really helps farmers make decisions and whether farmers readily assent to such technologies. What conditions must be met for acceptance? Are these conditions different if the information is used not only by farmers but also by other actors?
Introduction
Recent information and communication technologies (ICTs) have made exceptional advances in sensors, communications and data processing. There has been an exponential growth in the number of connected objects (around 15–20 billion connected objects in 2020, with a very strong increase (IDATE, 2017)), the consumer market being much larger than the professional market. The Internet of Things (IoT) has invested a growing number of farms. "The cow is the most connected animal in the world," says Nicolas Devos (Commercial Director of the IoT Agency at Open). What are the opportunities and threats of these new techniques for animal husbandry? Numerous reviews have been devoted to this topic for various livestock species (Wathes et al., 2008; Laca, 2009; Allain et al., 2014; Vranken and Berckmans, 2017; Fore et al., 2018; Grodkowski et al., 2018; Shalloo et al., 2018; Astill et al., 2020; Faverdin et al., 2020b; Lovarelli et al., 2020). The present review sets out to analyse the role of these new techniques in the chain from information acquisition to decision-making processes, in order to better identify risks and assets.
What to call the use of these new techniques in animal husbandry is still unsettled. “Precision livestock farming” is the term most commonly found in the literature. It is an extension of the term “precision farming”. However, is the aim of these techniques merely to allow more precision in the running of livestock farming systems? If we stick to the strict definition of “precision” – which implies correctness, accuracy or exactness –, probably not. Is precision a key element in a decision? Not necessarily. "Precision livestock farming" really implies close monitoring rather than accuracy. The term “made-to-measure” livestock farming has been proposed as an alternative to “precision livestock farming” (Médale, 2019), to emphasise that these new techniques offer means of adjusting livestock management to the needs of the animal, the objectives of the breeder, or the expectations of the consumer. “Made-to-measure” also stresses measures and thus data collected. One of the characteristics of these new techniques is certainly to provide more information, even if some of it is not very precise from a metrological point of view. Their purpose is to have rich, pre-processed information to refine livestock management. Reviews have already described the scope and applications of precision livestock farming in ruminants (Allain et al., 2014; Grodkowski et al., 2018; Shalloo et al., 2018).
This review will place emphasis on the information-decision relationship, which is at the core of how these new techniques are used. We will focus on examples in ruminant breeding. As shown in Figure 1, these new techniques bring innovative elements to all stages of the information-decision relationship in livestock farming. Sensors multiply the ways information can be acquired and transmitted, and databases increase the possibilities of organising and storing it. New computer learning methods or models increase capacity for information processing and decision support, or even decision-making capacities for carrying out operations in automated systems. Finally, these new techniques enable better traceability of operations, which can be automated and secure, as desired by the consumer. This overview will analyse the specific features of the new sources of information, which are richer and more diversified, available at greater frequency and for more entities. In the first section, we discuss the novel effects of enriching the information system. In the second section, we study how this information can help decision-making in animal husbandry. In decision theory, the term “information” means only what is likely to have an impact on the decision. Is this always the case? Does a plethora of information really help farmers improve the management of their farms and their work? What are the existing or potential uses of this mass of data? In the last section, we address the issue of the wider use of these new information systems. The information acquired for livestock farmers no longer seems to be intended solely for them. And what if added value were also to be made outside the livestock farm?
Figure 1. Insertion of new information and communication techniques (blue ovals) in the relations between information and decisions in livestock production systems.
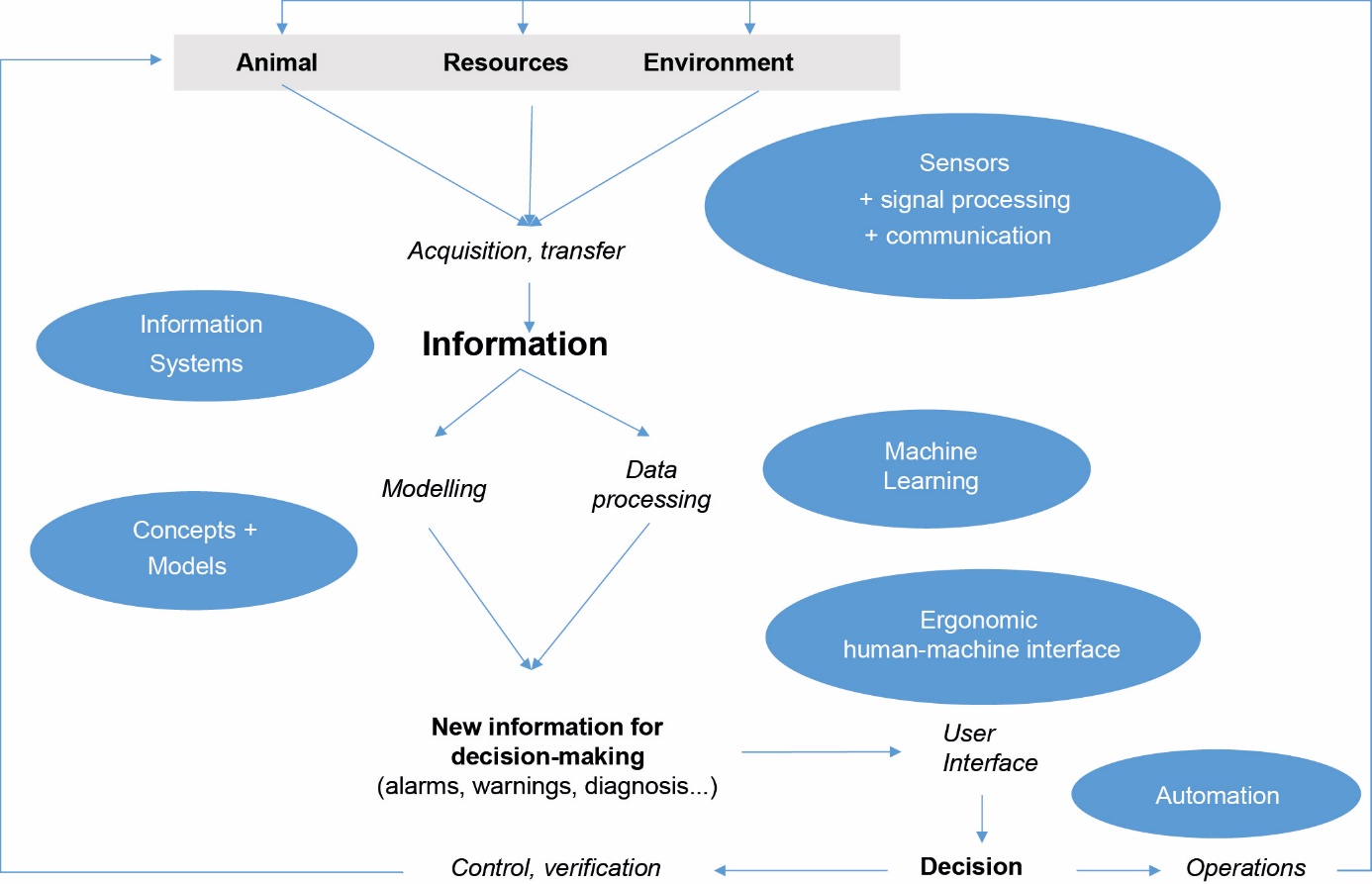
1. High throughput information
Acquiring information in animal husbandry is one of the farmer’s most important tasks. Such acquisition is very often dispersed over time and takes place during routine tasks (e.g. milking, feeding). It is essential to initiate actions. For instance, insemination of a female can only be carried out if the female is in heat, and a cow can only be treated if it is identified as sick. Information needs to be gathered not only on animals but also on equipment and buildings (is everything functioning properly – especially automated equipment?) and on food resources (feed stocks, grass growth), in order to repair malfunctions and manage resources. Assistance in acquiring information is potentially very helpful to farmers.
1.1. Automated acquisition of usual information in livestock farming
New information techniques have been designed to assist farmers in collecting various sorts of information they were already using, such as milk production or animal growth. The measurement of milk production (milk meters) and weighing (electronic scales) benefited from these new techniques very early on (Faverdin and Fischer, 2016). Similarly, for monitoring reproduction (detection of heat and, more recently, calving), sensors have proved very useful. We look here at the associated benefits.
The first benefit concerns the farmer's daily tasks. Farmers often report that carrying out these measurements or detections more or less automatically can reduce working time. This time-saving relies of course on the proper functioning of the devices. However, many measurement systems currently do not generate any malfunction alert, which implies that the farmer must still monitor the animals and these measuring devices. Monitoring in milk meters is generally well done (Allain et al., 2012), but that of other sensors is often haphazard. How do we detect that a collar with an accelerometer is no longer properly positioned or that one of the weighing sensors is no longer working properly? How are we informed that the transmission of data from sensors is faulty? Although it reduces some observation tasks, the use of information technologies generates a new type of work: monitoring and control of tools. It is thus important to develop devices that include their own control.
The second potential benefit lies in the improvement of the quality of information, which can be accompanied by an improvement in multi-performance. These new sensors sometimes make it possible to collect better information because monitoring is continuous and done at times when the farmer is unavailable, e.g. at night, which is of great importance for heat detection. High-frequency measurements can also detect events that might otherwise be overlooked. Thus a daily milk or live weight measurement is not just a more frequent measurement. Frequent measurement makes it possible to observe perturbations relative to normal through analysis of kinetics. The analysis of perturbations (Codrea et al., 2011) is a good indicator of animal stress (sanitary, thermal, behavioural) (Bareille et al., 2014). The analysis of the form, importance and concomitance of perturbations (Ben Abdelkrim et al., 2019) may even help to improve the quality of a diagnosis. Other variables can also be extrapolated from elementary variables. Modelling the kinetics of weight and daily milk can allow the estimation of intake and its perturbations (Faverdin et al., 2017), which is very difficult to measure directly.
1.2 New information, new phenotypes
The new sensors and the multiplicity of potentially measurable traits open up the possibility of obtaining increasingly broad and continuous knowledge of the phenotype of an animal or a herd, and of its environment.
a. The diversity of sensors, measurements and their processing
Until very recently, the available sensors were mainly dedicated to one measurement and/or the provision of one type of service. For example, physical-chemical sensors performing continuous measurements on animals or in livestock buildings: milk production, live weight, temperature, pH, gas or substance concentrations, milk component contents, etc. These sensors have the advantage of being easily controlled and verified with a metrological approach. Other types of sensor often require more complex processing to go from signal to information. This is the case for accelerometers (initially for heat detection), 3D imaging for body condition score (BCS), spectral analysis of samples (near or mid-infrared), or geolocation (radio frequency triangulation, GPS). For these devices, there are often no standards or specific metrological approaches. Moreover, the algorithm used to process the sensor data can change without the user even knowing, which complicates any possible qualification.
Equipment and services have gradually diversified as a result of technological progress, advances in data processing methods, and the pressure from end users for more information and lower investment costs. Some sensors such as accelerometers can now be used to alert farmers to health problems, feeding behaviour and calving, and to give indications on the levels of some animal welfare characteristics, in addition to detecting heat (Veissier et al., 2019). Some manufacturers are also moving towards innovative concepts to offer equivalent services, but at lower cost. For example, the use of video "tracking" to monitor animal behaviour is fast developing (Wurtz et al., 2019). This makes it possible to monitor several animals from a single piece of equipment and therefore at lower cost and so push back the limits of one-off observation. Similarly, the use of longer range identification devices (remote detection/identification techniques via Bluetooth Beacon or UHF RFID radio) makes it possible, for example, to detect the presence (or absence) of animals in key areas of the building (trough, drinking trough, cubicle, etc.) and thus provide individual information on their behaviour without the need for more expensive accelerometers. On the other hand, these techniques may be more dependent on the rearing conditions and require specific training in the system to associate places or positions with animal activity.
b. High throughput phenotyping
A very innovative feature of the new sensors is that they provide very frequent information on many animals. They also make it possible to observe traits that were previously less well recorded than the production traits and much harder to measure on large numbers of animals.
This new source of information is an asset for genetic selection. Today, phenotyping has become a greater sticking point for selection than genotyping, given the costs of the measurements. With these techniques, costs are lower and are often covered by the farmers, who use this information in their breeding management (automated heat detection, milk performance data, continuous weight, etc.). Farmers can also share this information with the entire profession if they wish to improve selection for breeding traits, as is already the case in particular for ketosis risks (Barbat-Leterrier et al., 2016). In addition, new traits may be available for breeding, such as efficiency and resilience (Phocas et al., 2014a).
For research, this makes it possible to extend the range of measurement techniques to all the functions and behaviour of animals to give an increasingly systemic vision of animal husbandry, "macroscopic" in the sense of De Rosnay (1975), which is of great help for studying complexity. Research in nutrition will have a much more comprehensive approach to the use of feed by animals to improve feed efficiency and control feeding (González et al., 2018). The study of traits related to health, reproduction, behaviour, food processing efficiency and the environment can be facilitated by new measurement tools, including on commercial farms, where previously information was mainly available only on production traits. Enabling multi-trait studies and a better understanding of the interactions between functions is likely to help research on animal adaptation and robustness, and on agroecology (Phocas et al., 2014b). All this should also help to better interpret all the data provided by these sensors. Today, research into these new techniques is lagging behind actual use on farms. Another challenge is the access to the data produced by these techniques (Egger-Danner et al., 2015). Data storage and accessibility are not homogeneous between the different technologies. For a given solution, there are usually as many systems, data formats and platforms as there are manufacturers. This may partly explain why it is still difficult to make gainful use of these data in decision-making.
2. How is this information used for decision support in animal husbandry?
In order to be useful to the farmer, information technologies must offer two major advantages: they must (i) alleviate or improve daily task management and (ii) improve the performance of livestock farming (production, health, reproduction, environment, animal welfare). Is this always the case? Faced with this mass of information, alerts and systems to be managed, how are farmers going to appropriate these new provisions to decide and manage their livestock? Is this information well-adapted to decision-making? Do farmers feel or have proof of a benefit from the introduction of these new techniques? How do they change their vision of the livestock farmer's profession? All these questions influence whether or not new techniques are adopted in animal husbandry.
2.1. Success stories: the case when the information was already being used in management
Certain techniques are currently very widespread on farms. For example, according to a survey conducted in France (Allain et al., 2015), nearly 70% of farmers are equipped with at least one connected tool. The most common ones are sensors for heat or calving detection (29%), and milking monitoring systems such as milk meters, conductivity meters or milk analysers (26%). Similar figures were reported among Dutch dairy farmers (Steeneveld and Hogeveen, 2015). Given the rapid growth of these technologies on farms, the equipment rate has probably increased significantly since these surveys were conducted. In most cases, these tools have been used to assist farmers in a monitoring or information acquisition activity that they were already carrying out by other means (e.g. by direct observation).
a. Identification and communication
For information to be usable over time, it is necessary both to know who it concerns and to transmit the data to a system that will use it to provide information to the decision-maker. Identification is often so implicit that we forget that it is the prerequisite for any action (Duroy, 2016). If the information relates to an animal, this animal must be identified. If the sensor is associated with an animal, it is sufficient to identify the sensor with the animal's identification number. This information should then be regularly transmitted to a database, if possible without user intervention. Unlike mobile phones (2G, 3G, 4G and 5G), the transmission of information at low speed with a long autonomy (up to 5–10 years with a small battery), a long range and low costs has also opened the way to communicating objects that become fully autonomous (Sigfox, Lora). Such systems do not need added wiring or computers and sometimes even no antenna: the connected object transmits its data (often stored in a "cloud") directly over the networks. The user no longer has to manage either collection or storage, which is greatly time-saving and also reduces the risk of information loss.
b. Automatic detection of heat and parturition
The reproduction of ruminants not only ensures the production of milk and meat on the farm, but also the renewal of the herd. The most essential reproduction events, such as heat and calving, must therefore be monitored precisely to ensure the best technical and economic performance of the livestock system. Heat can be observed visually by the farmer. However, this takes time – the current recommendation is to observe heat three times 15–20 min per day – and performance is limited (detection sensitivity of 50–60%). Avoiding dystocic calving and stillborn calves is also a priority, since the course of calving determines not only the birth of a viable calf, but also the health of the cow in the following weeks and its future reproductive performance. This surveillance is time-consuming and a source of stress for the farmer, even though it can sometimes be carried out remotely by video surveillance.
Automated detection systems for heat (activimeter, collar measuring activity and rumination, etc.), ovulation (on-line measurement of progesterone, etc.) and parturition (vaginal thermometer, activity sensor based on the tail) have been used on cattle farms (Mottram, 2016). They allow detection performance far superior to visual observations for heat (sensitivities of 60–100% for specificities above 90%) (Saint-Dizier and Chastant-Maillard, 2018). Nevertheless, many farmers further check these detections themselves before insemination and do not delegate the final decision to the sensor. For calving, the detection performance is excellent for accurately detecting the calf's expulsion. These devices often also make it possible to predict calving in the preceding days, so enabling farmers to anticipate and secure the calving.
While the performance of these techniques is undeniable, the benefits perceived by farmers are mainly in terms of workload and personal comfort (Disenhaus et al., 2016), although fears of loss of observation skills are often mentioned. For the detection of heat, besides the time saved by delegating surveillance to monitoring tools, it is the reduction in mental workload at the time of the decision to inseminate or not that is underlined. With regard to the detection of calving, the possibility of delegating monitoring to sensors during the night is often cited as a gain in personal comfort.
c. The measurement of milk production
The major innovation of automated individual measurement of milk production in livestock farming is no longer vaunted because the technology has now been routinely used for several decades and many dairy farms are now equipped with it. Dairy farmers wanted long ago to know individual milk yield and which cows were good vs. bad producers. Monthly milk recording still exists, but many farms are now equipped with milk meters. In the 2014 survey (Allain et al., 2015) already 10% of the farms were equipped with milk meters, to which is to be added the 13% of farms equipped with milking robots, and this figure must have risen considerably in the last six years. Measuring production at each milking provides more information than just the difference in production between cows. It can be used to detect problem animals or perturbations in herd management when there is a sudden drop in the production of an individual or the whole herd. It has become a tool for short-term herd management to be able to respond quickly to these problems. As mentioned in Section 1.1, production perturbations are good indicators of problems or anomalies, even if they do not allow precise diagnosis.
These examples show that the techniques that have replaced and automated often time-consuming methods of information acquisition soon found outlets on farms. These new techniques also offer new information that was not necessarily common on farms hitherto.
2.2. Information that needs to find a place in the decision-making process
As with smartphones and thanks to new computer tools for machine learning, more and more solutions are being proposed to extract information from very generic sensor data (accelerometer, GPS or image) where the raw data taken separately are not informative. We are witnessing an exponential development of precision livestock farming tools (generally sensors associated with data processing software), designed to monitor the health status of animals, their behaviour, their growth or their stress exposure (Veissier et al., 2019). To name but a few:
i) Real-time locating systems (RTLSs) to detect the position of animals and deduce their activity (e.g. CowView ®, CowManager ®, Smartbow ®) or accelerometers to detect whether an animal is standing, lying down, moving, eating or ruminating (e.g. Heat'Live ®, Time'Live ® and Feed'Live ®, IceQube ®); this data is interpreted to identify an animal in heat, sick, etc. (Wagner et al., 2020);
ii) motion detection cameras (e.g. Kinect ®) coupled with image analysis to measure the body condition or morphology of an animal, detect aggression, identify lesions, etc. (Fischer et al., 2015; Le Cozler et al., 2019; Lee et al., 2016);
iii) microphones to detect coughing or animal vocalisations (e.g. SoundTalks ®).
In general, algorithms are used to either classify observations (e.g. chicken feet with or without lesions) or to detect anomalies within time series. For example, the behaviour of an animal is first tracked to determine a baseline, and deviations from this baseline are then detected. These abnormalities are usually related to a reproductive event (heat, birth, etc.), a health problem (infectious disease, lameness, etc.) or a stress. These tools need to be validated in actual farm conditions: do they allow anomalies to be reliably detected and diagnosed? Very often, the performance in terms of sensitivity and specificity of detection is not stated. Detection is often limited to binary information and the question of the severity of the disorders is seldom addressed. As detection is often very early, the confirmation and identification of a disorder by clinical examination is not easy. Finally, there is a lack of a regulatory framework to specify minimum characteristics for these tools, including for safety.
There are many sensors that monitor health, but many of them were initially developed for the detection of calving or heat: for example, ear loops (Fevertags ®) or ruminal boluses (San'phone ®) measuring temperature (useful, for example, for the detection of respiratory disorders in bull breeding (Timsit et al., 2011)). Changes in rumination and activity alert the farmer to animals to be looked at more closely, without, however, giving a precise diagnosis of the condition in question. Finally, on milking robots, plentiful information related to udder health can be obtained (e.g. somatic cell count and milk conductivity), as the robot must detect mastitis and decide whether or not to discard the milk. Changes in milking frequency or milk production monitoring may indicate a health concern. New tools based on image analysis are being studied to detect animals with pain-expressing faces (Noor et al., 2020) and to identify behavioural perturbations. Pressure-sensing mats can be used to detect abnormalities in support and thus identify lameness. Again, as detection is often very early, confirmation and identification of a disorder by clinical examination is not easy. Furthermore, the reference or "gold standard" for assessing the sensitivity and specificity of the tool is easy to obtain for heat or calving, but much more difficult for health disorders, for instance the choice of validation windows (periods without alerts) has a large impact. If a calving alert is triggered, the observation of the event will enable this alert to be qualified. If the alert concerns the detection of heat, the comparison with a detection by the farmer, or a hormone assay will also allow it to be qualified. For health problems, the difficulties involve:
i) the alert itself: is the alert threshold fixed (e.g. T > 40 °C) or is it based on the animal taken as its own reference?
ii) the necessary period of recorded anomalies before generating the alert (sensitivity vs. specificity), and
iii) the "gold standard" to be used to qualify sensitivity and specificity. An alert based on hyperthermia or a drop in activity is in no way specific to a disease. It serves to identify animals that probably need a thorough examination, but it is not a diagnosis. The time window for validation of specificity (in the absence of an alert) is also problematic.
In addition to monitoring animals, information technology can help to manage resources, an application that is less frequently mentioned. However, this has significant impact on the environment (Faverdin and Brossard, 2019). The contribution of new techniques in precision feeding is eagerly awaited, but still a little disappointing. It seems that individualised feeding according to the needs defined from production allows a slight improvement in feed efficiency, all the more important as the nutrient intake before individualisation is high (Cutullic et al., 2013), but these practices complicate feed management. The most original approach, although its use is only moderately developed, is one in which the animal's response to concentrate intake is tested individually and levels are adjusted according to this response to achieve dynamic economic optimisation (André et al., 2010). It is undoubtedly in the use of nutritional supplements coupled with early detection of preclinical signs that a useful and original benefit of these techniques can be expected. Though more often mentioned in monogastric livestock farming, this aspect of resource management should not be neglected for ruminants to limit water or energy losses, and also to reduce nitrogen losses and optimise effluent management. Nevertheless, the offers are still weakly structured around this resource management, except for the control of buildings in pig or poultry production.
2.3. From decision support to delegation of the decision
While there are sensors whose information is not always easy to integrate into a decision, there are also techniques that are much more explicit in decision-making. However, is the farmer ready to delegate decision, or even action, to technology?
Most of the time, the techniques used by farmers are limited to the provision of information or alerts notifying the occurrence of a health disorder or breeding event. However, the aggregation of information from sensors with other information (economic, historical, technical) from livestock farming and recorded by other channels (performance monitoring, herd management software, etc.) would make it possible to produce targeted advice to facilitate a farmer's decision-making. The development of this type of decision support model by manufacturers represents a future prospect for precision farming. Some commercial systems already offer it.
The DeLaval Herd Navigator® (DeLaval), for example, which automatically performs enzyme determinations of milk parameters such as progesterone, lactate dehydrogenase or beta-hydroxybutyrate, uses the results of these determinations together with other individual information recorded by the farmer or measured by the milking robot to build a decision tree producing targeted advice. For example, for reproductive management, the system takes into account the parity of the cow, its lactation stage, or its production level in addition to the results of the progesterone test to advise the farmer whether and when to inseminate. The adoption of this automatic analysis system is still limited by its high cost, but its use allows the specific detection of ovulation, which offers better monitoring of reproduction than heat detection.
For precision feeding, the Dynamic Linear Model (DLM ®) was developed by Lely based on the model of André et al. (2010) in connection with its milking robots. This model automatically adjusts the concentrate distribution and milking frequency individually according to biological and technical considerations (production level, milk fat and protein content, milking interval and pre-milking time) and economic factors (milk price and feed cost) to optimise the economic margin per litre of milk and not only the production level. However, currently many farmers do not use this function, which can lead to unwelcome drops in production, but do use milking robot attendance control.
These two examples illustrate the fact that the level of delegation of the decision to robots by farmers is diverse. In addition, a lot of information is necessary for steering. However, most of the information used comes from measurements made by the milking robot or its peripherals, i.e. by tools coming mainly from the same system and the same manufacturer. In the future, the interoperability of tools should be ensured to enable the crossing of data from various sources and so improve the use of data for decision-making.
2.4. An uncertain cost/benefit ratio
New techniques make it possible in some cases to reduce workload by the automated collection of information by sensors, easier storage, rapid and centralised processing, decision-making support (feedback in the form of alerts or summary reports) and the possibility of focusing only on animals requiring special attention. However, these time savings are sometimes reduced if the herd is enlarged (Hostiou et al., 2017). The information produced by the sensors is also a source of stress, and sensors may not be adopted because they are too complex or too numerous (Russell and Bewley, 2013) (Table 1). These tools produce an abundance of information, making it difficult to select the information that will be useful for decision-making, leading to a high degree of selection. Only 3% of mastitis alerts from milking robots are reported to be actually used (Hogeveen et al., 2013). The information returned in the form of alerts is a cause of stress because it is too frequent and disrupts other farming activities. However, information can also reduce stress because farmers delegate the responsibility for detecting the event to the tool, particularly when physiological signs, such as heat, are more easily detected by sensors. For farmers, the new techniques also lend their profession a more modern image, making it more attractive (Faverdin et al., 2020b).
Table 1. Survey on obstacles to the adoption of new monitoring technologies in dairy farming.
Reason for low adoption rate |
% |
---|---|
1/ Not familiar with the available technologies |
55 |
2/ Undesirable cost/benefit ratio |
42 |
3/ Too much information provided without knowing what to do with it |
36 |
4/ Not enough time to spend with these technologies |
31 |
5/ Lack of perceived economic value |
30 |
6/ Too difficult or complex to use |
29 |
7/ Poor technical support or training |
28 |
(229 dairy farmers in Kentucky, US, according to Russell and Bewley, 2013)
Farmers often underline the gain in comfort and working time to justify investment in these techniques. Cost is still a barrier (Table 1). The overall cost depends on the cost of the equipment, the performance of the livestock farm before it was equipped, the farming system and how the farmer uses the techniques (Bekara and Bareille, 2019). When techniques are moderate in cost and produce information traditionally used (milk meters, heat or calving detectors, etc.), the return on investment is prompter than when they are expensive (milking or feeding robots) or provide information with which it is difficult to make a straightforward decision (e.g. monitoring health and welfare disorders). Uncertainty about the benefits of a sensor system and the expected improvements in herd management determine its adoption. This can explain why heat sensors are more readily adopted than sensors to measure body condition (Rutten et al., 2018). New marketing methods are emerging, which offer a package of services (Bouquet Farmlife, Medria Solutions) rather than specific equipment bought by farmers. Such services are likely to facilitate the assessment of potential benefits and the cost/benefit ratio by farmers. The future will tell whether this approach by services increases the adoption of ICT technologies by farmers.
2.5. From "technology-driven" to "decision-driven"?
Technology offers opportunities, but it takes time to use it intelligently. The goal is to move from the question, "What am I going to do with this new information?" to, "What information and with what qualities do I need to make better decisions on this or that aspect of management?" This move is very rarely made. It requires a much better understanding of the place of information in the decision-making. This is a very complex question, but it cannot be eluded if lasting improvements are to be made. A first option is to model the quality of the farmer's information system to see the effects on performance. For example, this approach has been tested on reproduction and has shown the impact on herd performance of the quality of health event detection and the specificity-sensitivity parameterisation of detection (Brun-Lafleur et al., 2010). Depending on the breeding system, the parameters and impacts differ.
By finely analysing how we make a good decision, we can revisit the question of information, sensors and their properties. In order to decide, it is essential to know what information is mobilised for a diagnosis, how decisions can be evaluated, what precision is needed for information and when it should be acquired. The use of models in decision-support tools led to a detailed discussion on the evaluation of these tools and the need to involve decision-makers in the process at a very early stage (Prost et al., 2012). The contribution of these new techniques in decision support plays a role very similar to that of models or expert systems. Do we make a better decision with this information? Are performances really improved? The evaluation of decision support tools based on these new techniques should be revisited. This will help to better qualify these tools so they will evolve towards better services for the farmer.
3. Information and certification: when the decision-maker is no longer necessarily the farmer
The data produced in a livestock farming system by all the connected objects and by the various automatic devices are now usually sent to large servers or "clouds" far from farms. Farmers generally benefit from the information or alerts generated by the processing of sensor data, but more rarely from the basic data, which is often of no interest to them. In this era of big data, it is clear that the data are not necessarily lost and can be used for other purposes. The question of sharing data generates some reticence. The use of these data, most of which are not covered by the General Data Protection Regulations (GDPRs), is governed by contractual relations. A number of proposals for charters have emerged over the last ten years in different countries to try to find acceptable compromises between farmers and potential users of their data. A comparison of these different charters has shown that they roughly cover the following aspects: (i) accessibility and readability of data, (ii) transparency of their use, (iii) control of their use, and (iv) data security (Hirschy, 2019).
3.1 Product traceability: guaranteeing specifications and results
Currently, data from various techniques are used "on site", for example in the management of a farm, transport unit or slaughterhouse, and are not exchanged between actors in the food chain. These data could inform a transport company as to whether an animal is fit for transport or not. They could also be used to compile information on welfare level over an animal’s life by integrating information from the farm, transport and slaughter. This information could then be shared with customers (processors, retailers) to diversify marketing or to provide guarantees to consumers.
The relationship that consumers/citizens have with agricultural products is radically changing. Historically, the need for security of supply and low prices were long the primary criteria. Quality and food safety were gradually added. It is no longer enough to merely ensure traceability within the sectors to quickly find the origin of a health problem. Today, expectations have become more complex and diversified, with a loss of confidence in production methods (environment, animal conditions, production system) (Delanoue et al., 2018). When buying or consuming a product, more and more consumers want to know where it comes from, how the animal was fed, treated, transported and slaughtered, how and where the product was processed, whether its production is environment-friendly and whether it has good nutritional qualities. Connecting the product to the farm and the producer is often enough to establish trust.
Some sectors already integrate data platforms or warehouses for their quality management and quality systems (e.g. De Hoeve Innovatie Group (KDV) in the Netherlands). Some use blockchain technology to link a product to the different locations it has passed through (farm, transport and slaughterhouse), adding information at each stage while securing this data and its origin to inform consumers (Connecting Food, Carrefour, etc.). This information could relate to environmental certification, compliance with specifications on animal feed (with minimum grazing, GMO-free, pesticide-free, urea-free, with omega-3, etc.), concern for animal welfare, etc. Figure 2 presents examples of segmentation of milk and meat products and the potential for using data from new techniques or other sources to check compliance with specifications.
Figure 2. Examples of possible segmentation of milk and meat products and sources of data that would allow objectification of compliance with specifications.
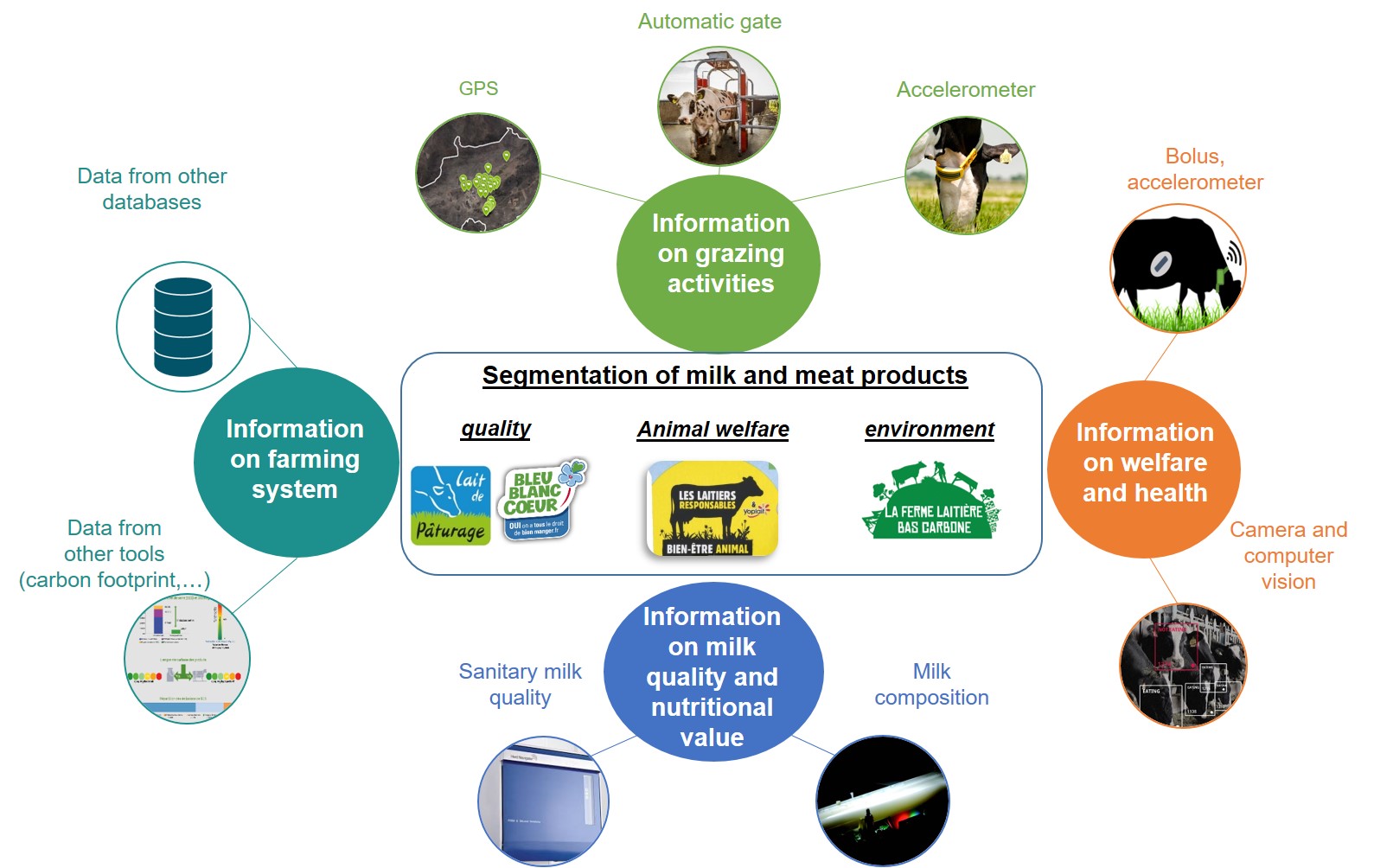
Such exchanges of information within the sectors require innovative business models, specifying who can do what with what data and ensuring fair sharing of costs and benefits between the actors of the agri-food chains.
3.2. The use of information for new applications
The connected "Internet of Things" paves the way for the reuse of information acquired in precision livestock farming system for purposes that tomorrow may be outside farms. Sophisticated computer systems are being developed to organise information brokerage between the various players (Vermesan and Friess, 2013). It is no longer necessarily a question of creating large databases that will gather all the information, but of managing information exchanges according to needs and applications. It is important to remember that when data are not linked to individuals and therefore do not fall within the scope of the DPMR framework, they are not owned. Outside this framework, only information systems can have ownership, but the owners may want to share this information in a contractual form depending on the purpose of this sharing. Different applications that concern livestock farming may offer new services based on the data collected and with the farmer’s consent.
Genetic selection requires the collection of much phenotypic information associated with the genetic information on the animals. The feedback of data to the selection centres would make it possible to propose new indices based on genomic information, as shown by the currently available genomic index on the risk of ketosis in dairy cows (Barbat-Leterrier et al., 2016). Parameters routinely recorded by automated milking robots, such as milking speed (Heringstad and Bugten, 2014), milk flow rates (Fogh et al., 2012), temperament (based on milking behaviour as measured by the number of times the milking clusters are unhooked (Rinell, 2013)), udder conformation (based on robot camera and laser beam recordings (Fogh et al., 2013), udder shape (based on robot camera and laser beam recordings (Fogh et al., 2012)) could easily be used for the selection of traits related to the milking ability of dairy cows.
It is possible to make better use of the possible synergies between the data. Applications could collect all the data of a farm to provide it with further information extracted from these data. This is the objective, for example, of the Applifarm company in France or JoinData in the Netherlands. Many data from monitoring can be used for instantaneous evaluation. However, there is great potential for wider use of these large databases, for example to help veterinarians diagnose problems and provide advice to producers. On an experimental basis for 18 months, French law No. 2020-526 of 5 May 2020 authorises the performance of veterinary acts by means of telemedicine, a field where IoT and the link with business software would be particularly useful. It is also possible to compare a farm with other farms in the same region by benchmarking. The analysis of these data over longer time steps can provide other indicators to help farmers in their decisions (integrated vision of health data, choices of reforms and reproduction, individualised management based on past information, etc.). The data generated by the IoT can allow the revisiting of norms considered physiological by taking each animal as its own control. The notion of health could be investigated by defining a set of indicators at the level of each animal. The current lack of clear and simple gateways between the software used by the various stakeholders (e.g. farmers and their veterinarians) limits the use of the data collected.
The "big data" approach to the large amount of data in agriculture has attracted much interest. Several projects in agriculture, especially in the livestock sector, will undertake to collect and process the masses of information (big data) resulting from new technologies in these sectors using new artificial intelligence technologies (Morota et al., 2018). Thus, the Microsoft "Farmbeats" initiative offers tools to achieve this (https://www.microsoft.com/en-us/research/project/farmbeats-iot-agriculture/). However, the combination of more mechanistic modelling approaches with big data approaches might well further improve the use of information for decision-making (Ellis et al., 2020). The stakes are high at the scale of dairy or meat sectors or territories to anticipate market situations, resource supply, health risks, etc. (Ellis et al., 2020). For example, in terms of health risks, algorithms continuously analysing the gestation periods of cows in syndromic surveillance (Marceau et al., 2014) would have made it possible to detect anomalies in northern France in 2008 only 7 weeks after the first clinical notification of bluetongue. Data collected continuously would make it possible, following the example of Google's famous Flu Trends algorithm, to detect the emergence of a new syndrome on a territorial scale.
Conclusion
ICT techniques facilitate the acquisition of information and allow access to much new information in animal husbandry. Some of these techniques are already widely used. This is only the beginning and further exciting applications will no doubt emerge. However, these techniques still require a significant amount of monitoring work to provide reliable information, an activity that is not part of the traditional tasks of farmers. Abundant information is provided by these technologies but their use in decision-making and management processes of livestock farming is not always clear. It is not enough to provide masses of data to assist in decision-making. Information must be relevant (few false alarms) and the farmer must know how to use it for livestock management. The most widespread applications currently meet these criteria. Before developing new applications, it will be advisable to find out what information farmers need most to help them in their decisions. Furthermore, the mass of data generated can find other applications than just in livestock management and many operators are interested in this. Traceability from farm to fork is already a reality and will be fuelled by this information (or will impose it); this will require finding a balance between the farmer's feeling of being controlled by other actors and the possibility of strengthening links between farmers and consumers.
Acknowledgments
This work was conducted with the support of the Digital Agriculture Convergence Lab #DigitAg (ANR-16-CONV-0004). This article was first translated with www.DeepL.com/Translator and the authors thank “Metaform Langues” for English language editing.
Notes
- This article was presented at the 25th Rencontres Recherches Ruminants (Faverdin et al., 2020a).
References
- Allain C., Trinderup M., Burke M., Rouzaut E., Sievert S., Schuiling E., Lassalas J., 2012. Computerized solutions for periodic checking of electronic milk meters. 38th ICAR Session, Cork. http://www.icar.org/wp-content/uploads/2015/09/Allain1.pdf
- Allain C., Chanvallon A., Clément P., Guatteo R., Bareille N., 2014. Élevage de précision : périmètre, applications et perspectives en élevage bovin. Renc. Rech. Rum., 21, 3-10.
- Allain C., Quinton P., Philibert A., Cros P., Herman M., Cimino M., Frappat B., Larsonneur S., Lafont N., 2015. La connectivité des élevages laitiers. Renc. Rech. Rum., 22, 403.
- André G., Berentsen P.B.M., Van Duinkerken G., Engel B., Lansink A., 2010. Economic potential of individual variation in milk yield response to concentrate intake of dairy cows. J. Agric. Sci., 148, 263-276.
- Astill J., Dara R.A., Fraser E.D.G., Roberts, B. Sharif, S., 2020. Smart poultry management: Smart sensors, big data, and the internet of things. Comput. Electron. Agric., 170, 105291.
- Barbat-Leterrier A., Leclerc H., Philippe M., Fritz S., Daviere J.B., Guillaume F., De Bretagne T., Boichard D., 2016. GénoSanté : améliorer la santé productive des vaches laitières par la sélection génomique et la conduite d’élevage : une première étape avec l’acétonémie. Renc. Rech. Rum., 23, 153-156.
- Bareille N., Videcoq L., Davière J.B., Johan M., Godin S., Leyrat-Bousquet E., Lemonnier J.P., Lamy J.M., Chanvallon A., 2014. Détection des troubles de santé des vaches laitières par la mesure de leur température ruminale. Renc. Rech. Rum., 21, 15-18.
- Bekara M.E.A., Bareille N., 2019. Quantification by simulation of the effect of herd management practices and cow fertility on the reproductive and economic performance of Holstein dairy herds. J. Dairy Sci., 102, 9435-9457.
- Ben Abdelkrim A., Puillet L., Gomes P., Martin O., 2019. Lactation curve model with explicit representation of perturbations as a phenotyping tool for dairy livestock precision farming. bioRxiv, 661249.
- Brun-Lafleur L., Rellier J.-P., Martin-Clouaire R., Faverdin P., 2010. Un simulateur de troupeau de vaches laitières avec modélisation du système d’information : application à la qualité de détection des chaleurs. Renc. Rech. Rum., 17, 257.
- Codrea M.C., Hojsgaard S., Friggens N.C., 2011. Differential smoothing of time-series measurements to identify disturbances in performance and quantify animal response characteristics: An example using milk yield profiles in dairy cows. J. Anim. Sci., 89, 3089-3098.
- Cutullic E., Delaby L., Edouard N., Faverdin P., 2013. Rôle de l’équilibre en azote dégradable et de l’alimentation protéique individualisée sur l’efficience d’utilisation de l’azote. Renc. Rech. Rum., 20, 53-56.
- Delanoue E., Dockès A.C., Roguet C., Magdelaine P., 2018. Les évolutions possibles de la controverse autour de l’élevage dessinent cinq futurs contrastés : Une analyse prospective à l’horizon 2040. Renc. Rech. Rum., 18, 510-519.
- De Rosnay J., 1975. Le macroscope. Vers une vision globale. Le Seuil, Paris. 346p.
- Disenhaus C., Quiniou Y., Allain C., Courties R., Bareille N., 2016. Discrepancy between expected and actual benefits of automatic heat detectors in commercial herds. 67th Annual Meeting of the European Federation of Animal Science 2016. Belfast. http://www.eaap.org/Annual_Meeting/2016_belfast/S69_10_Disenhaus.pdf
- Duroy S., 2016. Indentification électronique, capteurs embarqués, communication sans fil : voie d’entrée dans l’élevage de précision. In : Chastant-Maillard S., Saint-Dizier M. (Eds), Élevage de précision. Éditions France Agricole, Paris, France, 125-144.
- Egger-Danner C., Cole J.B., Pryce J.E., Gengler N., Heringstad B., Bradley A., Stock K.F., 2015. Invited review: overview of new traits and phenotyping strategies in dairy cattle with a focus on functional traits. Animal, 9, 191-207.
- Ellis J.L., Jacobs M., Dijkstra J., van Laar H., Cant J.P., Tulpan D., Ferguson N., 2020. Review: Synergy between mechanistic modelling and data-driven models for modern animal production systems in the era of big data. Animal, 14, S223-S237.
- Faverdin P., Brossard L., 2019. Efficience, agriculture de précision : de nouvelles approches environnementales ? In : Espagnol S., Brame C., Dourmad J.Y. (Eds). Pratiques d’élevage et environnement. Mesurer, évaluer, agir. Éditions Quae, Paris, France, 241-254.
- Faverdin P., Allain C., Guatteo R., Hostiou N., Veissier I, 2020a. Elevage de précision : De nouvelles informations utiles pour la décision ? Renc. Rech. Rum., 25, in press.
- Faverdin P., Brossard L., Hostiou N., 2020b. Le numérique au service de l’élevage : vers un élevage plus durable ? In : Chriki S., Ellies M.P., Hocquette J.F. (Eds). L’élevage pour l’agroécologie et l’alimentation durable. Éditions France Agricole, Paris, France, 157-175.
- Faverdin P., Fischer A., 2016. Monitoring du poids et de l'état corporel. In : Chastant-Maillard S., Saint,-Dizier M. (Eds). Élevage de précision. Éditions France Agricole, Paris, France, 125-144.
- Faverdin P., Charrier A., Fischer A., 2017. Prediction of dry matter intake of lactating dairy cows with daily live weight and milk production measurements, 8th Eur. Conf. Precision Livest. Farming (ECPLF), Nantes, France, 35-44.
- Fischer A., Luginbuhl T., Delattre L., Delouard J.M., Faverdin P., 2015. Rear shape in 3 dimensions summarized by principal component analysis is a good predictor of body condition score in Holstein dairy cows. J. Dairy Sci., 98, 4465-4476.
- Fogh A., Lauritsen U., Aamand G.P., 2012. Use of data from electronic milk meters and perspectives in use of other objective measures. 38th ICAR Ann. Meet., Cork, Ireland. http://www.icar.org/Cork_2012/Manuscripts/Published/Fogh.pdf
- Fore M., Frank K., Norton T., Svendsen E., Alfredsen J.A., Dempster T., Eguiraun H., Watson W., Stahl A., Sunde L.M., Schellewald C., Skoien K.R., Alver M.O., Berckmans D., 2018. Precision fish farming: A new framework to improve production in aquaculture. Biosyst. Eng. 173, 176-193.
- González L., Kyriazakis I., Tedeschi L., 2018. Review: Precision nutrition of ruminants: Approaches, challenges and potential gains. Animal, 12, S246-S261.
- Grodkowski G., Sakowski T, Puppel K., Baars T., 2018. Comparison of different applications of automatic herd control systems on dairy farms-a review. J. Sci. Food Agric., 98, 5181-5188.
- Heringstad B., Bugten H.K., 2014. Genetic evaluations based on data from automatic milking systems. In 39th ICAR annual meeting, Berlin Germany, http://www.icar.org/Documents/Berlin_2014/PPTs/Presented/Heringstad.pdf.
- Hirschy G., 2019. Multipass. Episode « Panorama des chartes d’utilisation et de partage des données agricoles ». https://numerique.acta.asso.fr/multipass-5-charte/
- Hogeveen H., Buma K.J., Jorritsma R., 2013. Use and interpretation of mastitis alerts by farmers. In: Proc. 6th Eur. Conf. Precision Livest. Farming. Berckmans D., Vandermeulen J. (Eds). Leuven, Belgique, 313- 319.
- Hostiou N., Fagon J., Chauvat S., Turlot A., Kling-Eveillard F., Boivin X., Allain C., 2017. Impact of precision livestock farming on work and human-animal interactions on dairy farms. A review. Biotechnol. Agron. Soc. Environ., 21, 268-275.
- IDATE, 2017. https://fr.idate.org
- Laca E.A., 2009. Precision livestock production: tools and concepts. Rev. Bras. Zootec., 38,123-132.
- Le Cozler Y., Allain C., Caillot A., Delouard J.M., Delattre L., Luginbuhl T., Faverdin, P., 2019. High-precision scanning system for complete 3D cow body shape imaging and analysis of morphological traits. Comput. Electron. Agric., 157, 447-453.
- Lee J., Jin L., Park D., Chung Y., 2016. Automatic Recognition of Aggressive Behavior in Pigs Using a Kinect Depth Sensor. Sensors, 16, 631.
- Lovarelli D., Bacenetti J., Guarino M., 2020. A review on dairy cattle farming: Is precision livestock farming the compromise for an environmental, economic and social sustainable production. J. Cleaner Prod., 262, 121409.
- Marceau A., Madouasse A., Lehébel A., van Schaik G., Veldhuis A., Van der Stede Y., Fourichon C., 2014. Can routinely recorded reproductive events be used as indicators of disease emergence in dairy cattle? An evaluation of 5 indicators during the emergence of bluetongue virus in France in 2007 and 2008. J. Dairy Sci., 97, 6135-6150.
- Médale F., 2019. Élevage sur mesure : des innovations au service de l’animal, de l’éleveur et du consommateur. INRAE. Plaquette « Innovez avec l’INRA sur... ».
- Morota G., Ventura R.V., Silva F.F., Koyama M., Fernando S.C., 2018. Big data analytics and precision animal agriculture symposium: Machine learning and data mining advance predictive big data analysis in precision animal agriculture. J. Anim. Sci., 96, 1540-1550.
- Mottram T., 2016. Animal board invited review: precision livestock farming for dairy cows with a focus on oestrus detection. Animal, 10, 1575-1584.
- Noor A., Zhao Y.Q., Koubaa A., Wu, L.W., Khan R., Abdalla, F.Y.O., 2020. Automated sheep facial expression classification using deep transfer learning. Comput. Electron. Agric., 175. 105528.
- Phocas F., Agabriel J., Dupont-Nivet M., Geurden I., Médale F., Mignon-Grasteau S., Gilbert H., Dourmad J.Y., 2014a. Le phénotypage de l’efficacité alimentaire et de ses composantes, une nécessité pour accroître l’efficience des productions animales. INRA Prod. Anim., 27, 235-247.
- Phocas F., Bobe J., Bodin L., Charley B., Dourmad J.Y., Friggens N.C., Hocquette J.F., Le Bail P.Y., Le Bihan-Duval E., Mormède P., Quéré P., Schelcher F., 2014b. Des animaux plus robustes : un enjeu majeur pour le développement durable des productions animales nécessitant l’essor du phénotypage fin et à haut débit. In : Phocas F. (Ed). Phénotypage des animaux d’élevage. INRA Prod. Anim., 27, 181-194.
- Prost L., Cerf M., Jeuffroy M.H., 2012. Lack of consideration for end-users during the design of agronomic models. A review. Agron. Sustainable Dev., 32, 581-594.
- Rinell E., 2013. A genetic analysis of traits recorded by automatic milking systems - the possibility for a new method to evaluate temperament of dairy cows. Thèse Master, Uppsala, 24p. http://stud.epsilon.slu.se/5967/7/rinell_e_130821.pdf
- Russell R.A., Bewley J.M., 2013. Characterization of Kentucky dairy producer decision-making behavior. J. Dairy Sci., 96, 4751-4758.
- Rutten C.J., Steeneveld W., Lansink A., Hogeveen H., 2018. Delaying investments in sensor technology: The rationality of dairy farmers' investment decisions illustrated within the framework of real options theory. J. Dairy Sci., 101, 7650-7660.
- Saint-Dizier M., Chastant-Maillard S., 2018. Potential of connected devices to optimize cattle reproduction. Theriogenology, 112, 53-62.
- Shalloo L., O' Donovan M., Leso L. Werner J., Ruelle E., Geoghegan A., Delaby L., O'Leary N., 2018. Review: Grass-based dairy systems, data and precision technologies. Animal, 12, S262-S271.
- Steeneveld W., Hogeveen H., 2015. Characterization of Dutch dairy farms using sensor systems for cow management. J. Dairy Sci., 98, 709-717.
- Timsit E., Assie S., Quiniou R., Seegers H., Bareille N., 2011. Early detection of bovine respiratory disease in young bulls using reticulo-rumen temperature boluses. Vet. J., 190, 136-142.
- Veissier I., Kling-Eveillard F., Mialon M.M., Silberberg M., De Boyer Des Roches A., Terlouw C., Ledoux D., Meunier B., Hostiou N., 2019. Élevage de précision et bien-être en élevage : la révolution numérique de l’agriculture permettra-t-elle de prendre en compte les besoins des animaux et des éleveurs ? INRA Prod. Anim., 32, 281-290.
- Vermesan O., Friess P., 2013. Internet of Things: Converging Technologies for Smart Environments and Integrated Ecosystems. River Publishers, Aalborg, Denmark, 348p.
- Vranken E., Berckmans D., 2017. Precision livestock farming for pigs. Anim. Front., 7, 32-37.
- Wagner N., Antoine V., Mialon M.-M., Lardy R., Silberberg M., Koko J., Veissier I., 2020. Machine learning to detect behavioural anomalies in dairy cows under subacute ruminal acidosis. Comput. Electron. Agric., 170, 105233.
- Wathes C.M., Kristensen H.H., Aerts J.M., Berckmans D., 2008. Is precision livestock farming an engineer's daydream or nightmare, an animal's friend or foe, and a farmer's panacea or pitfall? Comput. Electron. Agric., 64, 2-10.
- Wurtz K., Camerlink I., D'Eath R.B., Fernandez A.P., Norton T., Steibel J., Siegford J., 2019. Recording behaviour of indoor-housed farm animals automatically using machine vision technology: A systematic review. Plos One, 14, e0226669.
Abstract
New technologies for sensors, communication and data processing have entered farms. This new source of information is more diverse, obtained at higher frequencies, for longer periods of time and from more entities. They raise new problems of qualification, reliability and maintenance of information, which remain largely to be explored. In decision theory, ’information’ relates to what is likely to have an impact on a decision. When the information is acquired by automatic devices rather than obtained by observation or measurement by humans, such devices quickly find their place on the condition that they are reliable. Information on animal health and welfare or on the environment, resources are still little used and their integration into effective decision-making tools is still insufficient. In addition, today, the use of this information is not limited to the livestock farm. Consumers want to know where their food comes from, how it was produced, with what level of environment protection or animal welfare, and to be able to trust this information. In conclusion, new information technologies allow accessing to a lot of new information in animal production, but it is not yet clear how it can be used in the decision-making by livestock farmers or other actors of the food-chain.
Attachments
No supporting information for this article##plugins.generic.statArticle.title##
Views: 11775
Downloads
PDF: 467
XML: 109
Most read articles by the same author(s)
- Mohamed Taher SRAÏRI, Vincent CHATELLIER, Christian CORNIAUX, Bernard FAYE, Claire AUBRON, Nathalie HOSTIOU, Alejandra SAFA, Saïd BOUHALLAB, Sylvie LORTAL, Sustainability of dairy development: reflections on a few cases in the world , INRAE Productions Animales: Vol. 32 No. 3 (2019)
- Isabelle VEISSIER, Florence KLING-EVEILLARD, Marie-Madeleine MIALON, Mathieu SILBERBERG, Alice DE BOYER DES ROCHES, Claudia TERLOUW, Dorothée LEDOUX, Bruno MEUNIER, Nathalie HOSTIOU, Precision Livestock Farming and animal welfare: is the numerical revolution of agriculture able to take into account animals’ and farmers’ needs? , INRAE Productions Animales: Vol. 32 No. 2 (2019): Volume 32 Issue 2: Special Issue. Major challenges and solutions for livestock farming
- Jean-Louis PEYRAUD, Joël AUBIN, Marc BARBIER, René BAUMONT, Cécile BERRI, Jean-Pierre BIDANEL, Christine CITTI, Corinne COTINOT, Christian DUCROT, Pierre DUPRAZ, Philippe FAVERDIN, Nicolas FRIGGENS, Sabine HOUOT, Marie-Odile NOZIÈRES-PETIT, Claire ROGEL-GAILLARD, Véronique SANTÉ-LHOUTELLIER, Science for tomorrow’s livestock farming: A forward thinking conducted at INRA , INRAE Productions Animales: Vol. 32 No. 2 (2019): Volume 32 Issue 2: Special Issue. Major challenges and solutions for livestock farming
- Gérard SERVIÈRE, Sophie CHAUVAT, Nathalie HOSTIOU, Sylvie COURNUT, Work in Livestock and its changes , INRAE Productions Animales: Vol. 32 No. 1 (2019)
- Gonzalo CANTALAPIEDRA-HIJAR, Philippe FAVERDIN, Nicolas C FRIGGENS, Pauline MARTIN, Feed Efficiency: towards a better understanding of it as a key element of sustainable livestock systems , INRAE Productions Animales: Vol. 33 No. 4 (2020)
- Caroline DEPOUDENT, Nathalie HOSTIOU, Lisa LE CLERC, Working in pig farming: attractiveness factors and constraints , INRAE Productions Animales: Vol. 34 No. 2 (2021)
- Philippe FAVERDIN, Jaap VAN MILGEN, Integrate scale changes to improve the efficiency of livestock production (systems?) and reduce emissions , INRAE Productions Animales: Vol. 32 No. 2 (2019): Volume 32 Issue 2: Special Issue. Major challenges and solutions for livestock farming
- Dominique POMIÈS, Caroline CONSTANCIS, Julien JURQUET, Isabelle VEISSIER, Hugues CAILLAT, Gilles LAGRIFFOUL, Marie DROUET, Clément FOSSAERT, Yannick LE COZLER, The future of young dairy ruminants: how to conciliate livestock farming and societal expectations , INRAE Productions Animales: Vol. 36 No. 1 (2023)
- Isabelle VEISSIER, Véronique DEISS, Ethics and legislation in animal experimentation in France: Food from thought from experiments on cattle , INRAE Productions Animales: Vol. 35 No. 2 (2022)
- Christian DUCROT, Maria Belén BARRIO, Alain BOISSY, François CHARRIER, Sergine EVEN, Pierre MORMÈDE, Sandrine PETIT, Marie-Hélène PINARD-VAN DER LAAN, François SCHELCHER, François CASABIANCA, Alain DUCOS, Gilles FOUCRAS, Raphaël GUATTEO, Jean-Louis PEYRAUD, Muriel VAYSSIER-TAUSSAT, Patrick VEYSSET, Nicolas C. FRIGGENS, Xavier FERNANDEZ, Jointly improving animal health and welfare in the transition of livestock systems towards sustainability , INRAE Productions Animales: Vol. 37 No. 3 (2024)